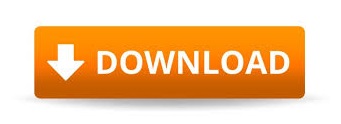
In total he generated 175 million positions in this way. He then created greater variety by adding a random legal move to each position before using it for training. Lai generated his dataset by randomly choosing five million positions from a database of computer chess games. Use a dataset that is too small and the network can settle into a state that fails to recognize the wide variety of patterns that occur in the real world. The massive number of connections inside a neural network have to be fine-tuned during training and this can only be done with a vast dataset. That’s because although unequal positions rarely arise in real chess games, they crop up all the time in the searches that the computer performs internally.Īnd this data set must be huge. It must also have plenty of variety of unequal positions beyond those that usually occur in top level chess games. “For example, it doesn’t make sense to train the system on positions with three queens per side, because those positions virtually never come up in actual games,” he says. This data set must have the correct distribution of positions. Lai trains his network with a carefully generated set of data taken from real chess games. The second looks at piece-centric features such as the location of each piece on each side, while the final aspect is to map the squares that each piece attacks and defends. The first looks at the global state of the game, such as the number and type of pieces on each side, which side is to move, castling rights and so on. His network consists of four layers that together examine each position on the board in three different ways. So it’s no surprise that deep neural networks ought to be able to spot patterns in chess and that’s exactly the approach Lai has taken. These so-called deep neural networks have become hugely powerful and now routinely outperform humans in pattern recognition tasks such as face recognition and handwriting recognition. That has allowed computer scientists to train much bigger networks organized into many layers. The second is the availability of massive annotated datasets to train the networks.
#PLAY DEEP BLUE CHESS HOW TO#
The first is a better understanding of how to fine-tune these networks as they learn, thanks in part to much faster computers. In the last few years, neural networks have become hugely powerful thanks to two advances. Lai has created an artificial intelligence machine called Giraffe that has taught itself to play chess by evaluating positions much more like humans and in an entirely different way to conventional chess engines. That dramatically simplifies the computational task because it prunes the tree of all possible moves to just a few branches.Ĭomputers have never been good at this, but today that changes thanks to the work of Matthew Lai at Imperial College London. This trick is in evaluating chess positions and narrowing down the most profitable avenues of search. Clearly, humans have a trick up their sleeve that computers have yet to master. And yet he played at essentially the same level. While Deep Blue was searching some 200 million positions per second, Kasparov was probably searching no more than five a second. Of course, no human can match that or come anywhere close. Their power relies on brute force, the process of searching through all possible future moves to find the best next one. Since then, chess-playing computers have become significantly stronger, leaving the best humans little chance even against a modern chess engine running on a smartphone.īut while computers have become faster, the way chess engines work has not changed. It’s been almost 20 years since IBM’s Deep Blue supercomputer beat the reigning world chess champion, Garry Kasparov, for the first time under standard tournament rules.
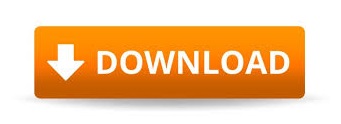